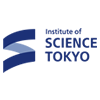
CLS
Laboratory for Chemistry and Life Science, Institute of Integrated Research, Institute of Science Tokyo
東京科学大学
総合研究院
化学生命科学研究所
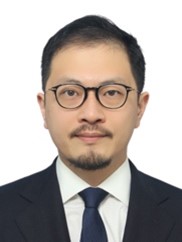
LAST UPDATE 2024/08/27
-
研究者氏名
Researcher Name安藤康伸 Yasunobu ANDO
准教授 Associate Professor -
所属
Affiliation東京科学大学 総合研究院 化学生命科学研究所
分子先駆化学領域
Laboratory for Chemistry and Life Science, Institute of Integrated Research, Institute of Science Tokyo
Molecular Advanced Chemistry -
研究キーワード
Research Keywords計算物質科学
マテリアルズ・インフォマティクス
表面・界面科学
研究D X
Computational Materials Science
Materials Informatics
Surface and Interface Science
Research Digital Transformation
- 研究テーマ
Research Subject -
データ駆動材料研究に向けた計算・情報基盤技術の開発
Development of infrastructure technologies for data-driven materials research
研究の背景 Background of the Research
材料科学と情報科学を組み合わせることで研究・開発速度を加速させ、さらに質的にも異なる研究を目指す試みであるマテリアルズ・インフォマティクスは2010年代より注目を集めています。2020年代に入るとこの流れはさらに激しくなり、ロボティクスと組み合わせた自立自動実験装置や、機械学習による新物質探索などが多数報告されており、材料科学を新しい段階に進めることが期待されています。
Materials informatics, an attempt to combine materials science and informatics in order to accelerate the R&D and to aim for more qualitatively different research, has been attracting attention since the 2010s. In the 2020s, this trend has intensified, with many reports of autonomous experimental systems and the search for new materials through machine learning. This is expected to take materials science to a new stage.
研究の目標 Research Objective
私たちのグループでは、データ生成・データ蓄積・データ活用の3つのフェーズに対応した基盤技術開発とその応用を目指します。特に、自動実験のためのソフトウェア開発や機械学習ポテンシャルを用いた物質シミュレーション、そしてハイスループットスペクトル解析技術などを活用し、蓄電材料・触媒材料・電子材料等に対するデータ駆動型材料研究の進展に貢献します。
Our group aims to develop and apply infrastructure technologies for the three phases of data generation, data accumulation and data analysis. In particular, through developing software for automated experiments, materials simulation using machine learning potentials, and high-throughput spectral analysis technology, we will contribute to the advancement of data-driven materials research for energy storage, catalytic and electronic materials.
研究図Figures
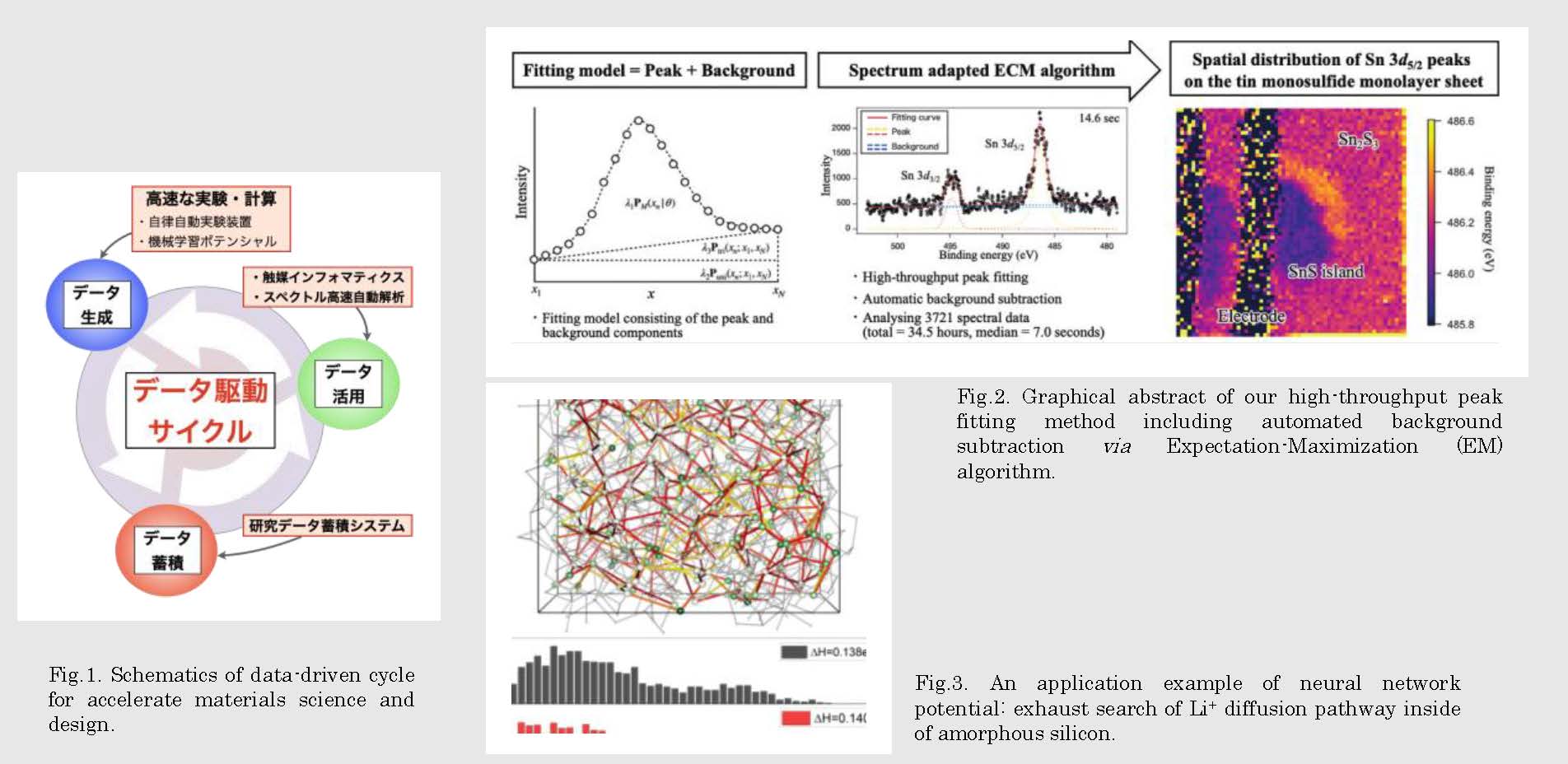
Fig.2. Graphical abstract of our high-throughput peak fitting method including automated background subtraction via Expectation-Maximization (EM) algorithm.
Fig.3. An application example of neural network potential: exhaust search of Li+ diffusion pathway inside of amorphous silicon.
論文発表 / Publications
[1] High-Throughput XPS Spectrum Modeling with Autonomous Background Subtraction for 3 d 5/2 Peak Mapping of SnS, Sci. Technol. Adv. Mater. Meth. 3, 2159753 (2023).[2] Autonomous Materials Synthesis by Machine Learning and Robotics, APL Materials 8, 111110 (2020). [3] High-Dimensional Neural Network Atomic Potentials for Examining Energy Materials: Some Recent Simulations, J. Phys. Energy 3, 012003 (2021). [4] 研究・技術の現場でAIを活用するためのはじめの一歩, 砥粒加工学会誌 65, 175 (2021).
研究者連絡先 / HP
- ando.y.am
m.titech.ac.jp、andou.y.1355
m.isct.ac.jp
- https://www.cd-mach.cls.iir.titech.ac.jp/activity/ando-activity