SANKEN(ISIR)
The Institute of Scientific and Industrial Research, Osaka University
大阪大学
産業科学研究所
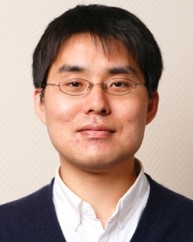
LAST UPDATE 2017/11/01
-
研究者氏名
Researcher Name原聡 Satoshi HARA
助教 Assistant Professor -
所属
Professional Affiliation大阪大学産業科学研究所
第一研究部門・知能推論研究分野
The Institute of Scientific and Industrial Research, Osaka University
Department of Reasoning for Intelligence -
研究キーワード
Research Keywords機械学習
データマイニング
人工知能
Machine Learning
Data Mining
Artificial Intelligence
- 研究テーマ
Research Subject -
機械学習モデルの解釈性向上
Improving Interpretability of Machine Learning Models
研究の背景 Background
機械学習を代表とする人工知能技術の発展により、我々の日常生活にも人工知能技術が徐々に浸透しつつある。近年の機械学習モデルは非常に複雑な構造をしており、内部でデータから何を読み取りどのような基準でもって判断を下しているからを人間が知ることはできない。ランダムフォレスト(数百本の決定木を組み合わせるモデル)や深層ニューラネット(多数のネットワークを層状に重ねたモデル)はこのような複雑なモデルの代表例である。現在の技術では、これらの複雑なモデルに判断の根拠を提示させることはできない。
Artificial Intelligence technologies such as machine learning are now getting ubiquitous in our daily lives. Most of popular machine learning models have very complex structure and it is therefore almost impossible for human to interpret the internal mechanism of the models. The examples are random forest, which is an ensemble of hundreds of decision trees, and deep neural networks, which consists of several network layers. These models are complete black-box, and it does not describe any reasons on their decisions.
研究の目標 Outcome
本研究の目標は、ランダムフォレストや深層ニューラルネットといった複雑なブラックボックスモデルに、その出力に関する根拠を提示させることでモデルの解釈性を向上させることである。ランダムフォレストの解釈性向上には、そのモデルの内部的な構造を一度分解して、人間が理解しやすいように再構成する方法が有用である。現在は、効果的かつ計算効率的な再構築方法の研究を進めている。これにより、従来ブラックボックスとされていたランダムフォレスの内部的な判断根拠を人間に明示的に伝えることができるようになる。
We aim at improving interpretability of complex machine learning models, such as random forest and deep neural network, by making their decision reasons to be transparent to human. In our current research, we focus on factorizing random forests into its building blocks and then reconstructing them so that the model to be interpretable. Specifically, we are working on developing effective and computationally efficient reconstruction algorithms.
研究図Research Figure
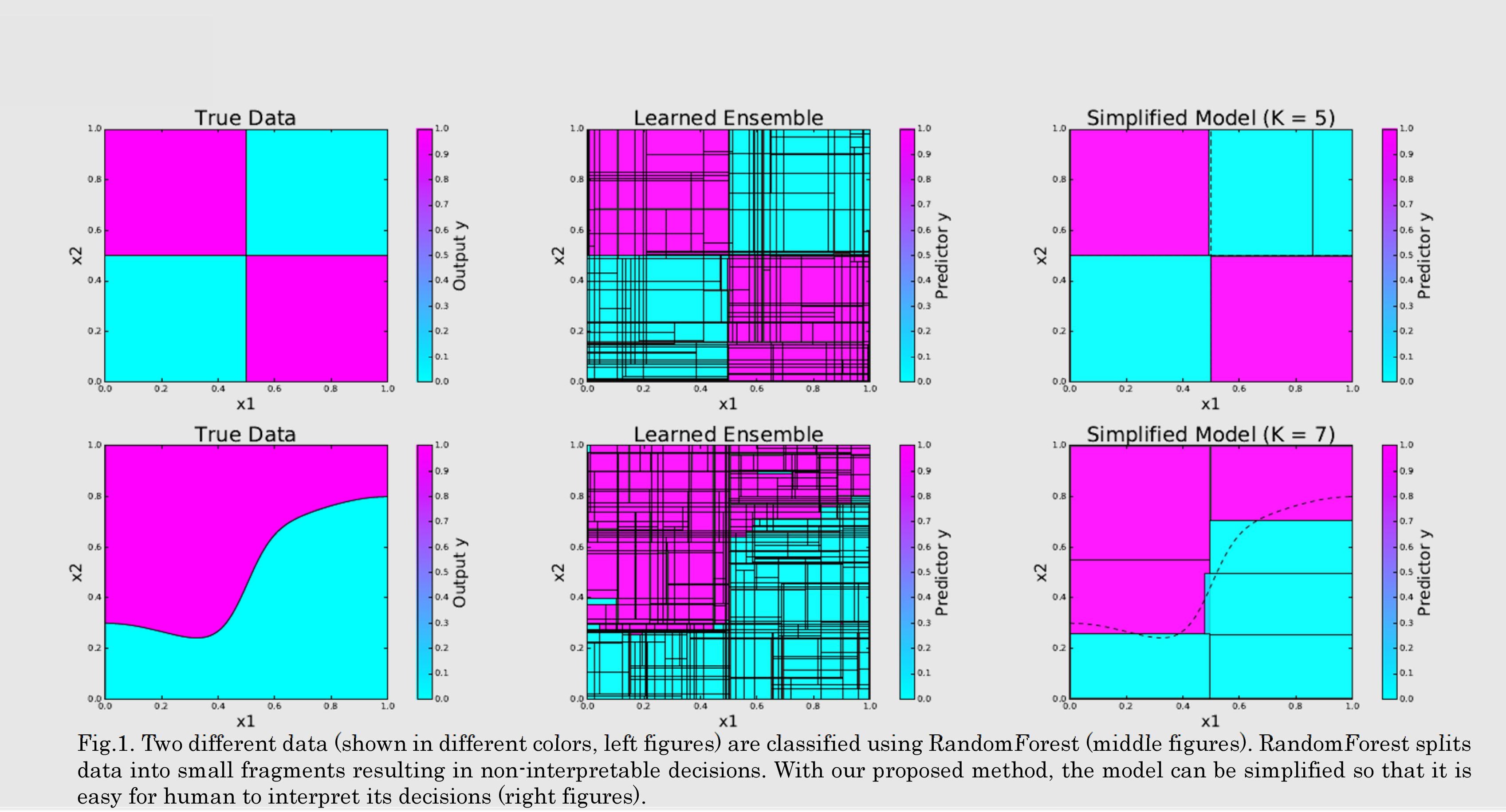
文献 / Publications
[1] Satoshi Hara and Kohei Hayashi. Making Tree Ensembles Interpretable: A Bayesian Model Selection Approach. arXiv:1606.09066, 2016.
[2] Satoshi Hara and Kohei Hayashi. Making Tree Ensembles Interpretable. In Proceedings of the 2016 ICML Workshop on Human Interpretability in Machine Learning, pages 81--85, 2016.
研究者HP
- satohara
sanken.osaka-u.ac.jp
- https://sites.google.com/site/sato9hara/