SANKEN(ISIR)
The Institute of Scientific and Industrial Research, Osaka University
大阪大学
産業科学研究所
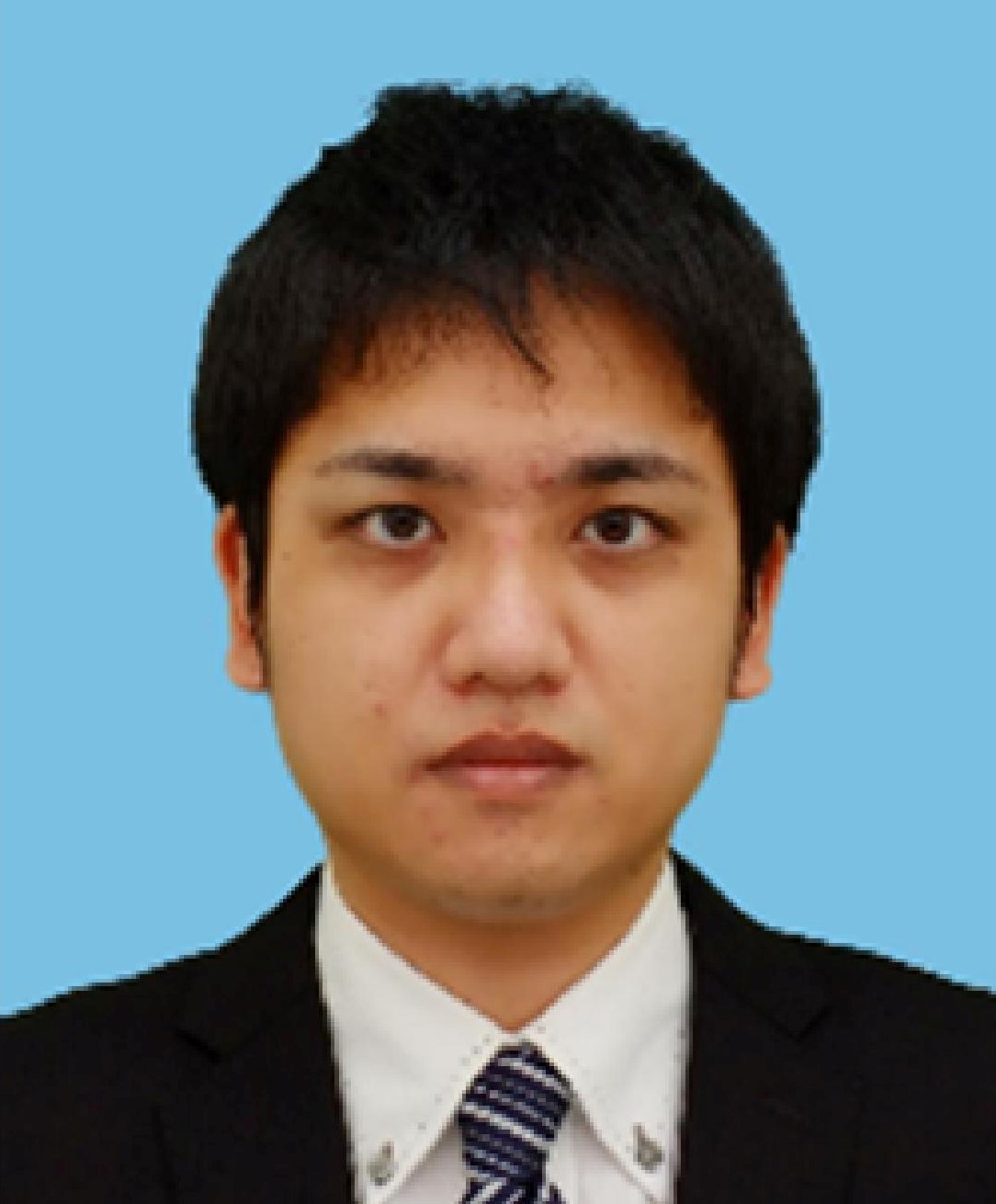
LAST UPDATE 2021/06/15
-
研究者氏名
Researcher Name山下泰信 Yasunobu YAMASHIATA
助教 Assistant Professor -
所属
Professional Affiliation大阪大学産業科学研究所
複合分子化学研究分野
The Institute of Scientific and Industrial Research, Osaka University
Department of complex molecular chemistry -
研究キーワード
Research Keywords創薬化学
有機化学
Medicinal Chemistry
Organic Chemistry
- 研究テーマ
Research Subject -
深層学習を用いた新規活性予測期の開発と応用
Development of activity predictor using deep learning and its application
研究の背景 Background
機械学習は様々な分野で功績をあげていますが、創薬分野においては汎用的な方法論は未だ確立されていません。その理由は、化学構造の[入力]に熟練した研究者の豊富な経験を元にした、記述子の選択が必要であることと考えています。そこで、AI自身に化合物全体を学習させる深層学習を用いた薬物スクリーニングに注目しました。
Machine learning has been successful in many fields, but no general methodology has yet been established in the medicinal field. This reason is that it is necessary to select a descriptor based on the experience of researchers to input of chemical structures. Therefore, drug screening using deep learning, which allows AI itself to learn the entire compound is focused.
研究の目標 Outcome
深層学習を利用し高い活性化合物を予測する創薬方法論を開発し、これまで見出すことができなかった新規骨格を持つ生理活性物質を発見することを目標としています。構造式から記述子への変換プロセスを経由せずに化学構造自体を「入力」し、AI自身に化合物全体を学習させる深層学習を用いています。
The purpose of this research is to develop a drug discovery methodology that predicts highly active compounds using deep learning, and to discover bioactive substances with a novel structure. In this research, deep learning is utilized to input the chemical structure itself without the process of converting structural formulas to descriptors, enabling the AI itself to learn the entire compound.
研究図Research Figure
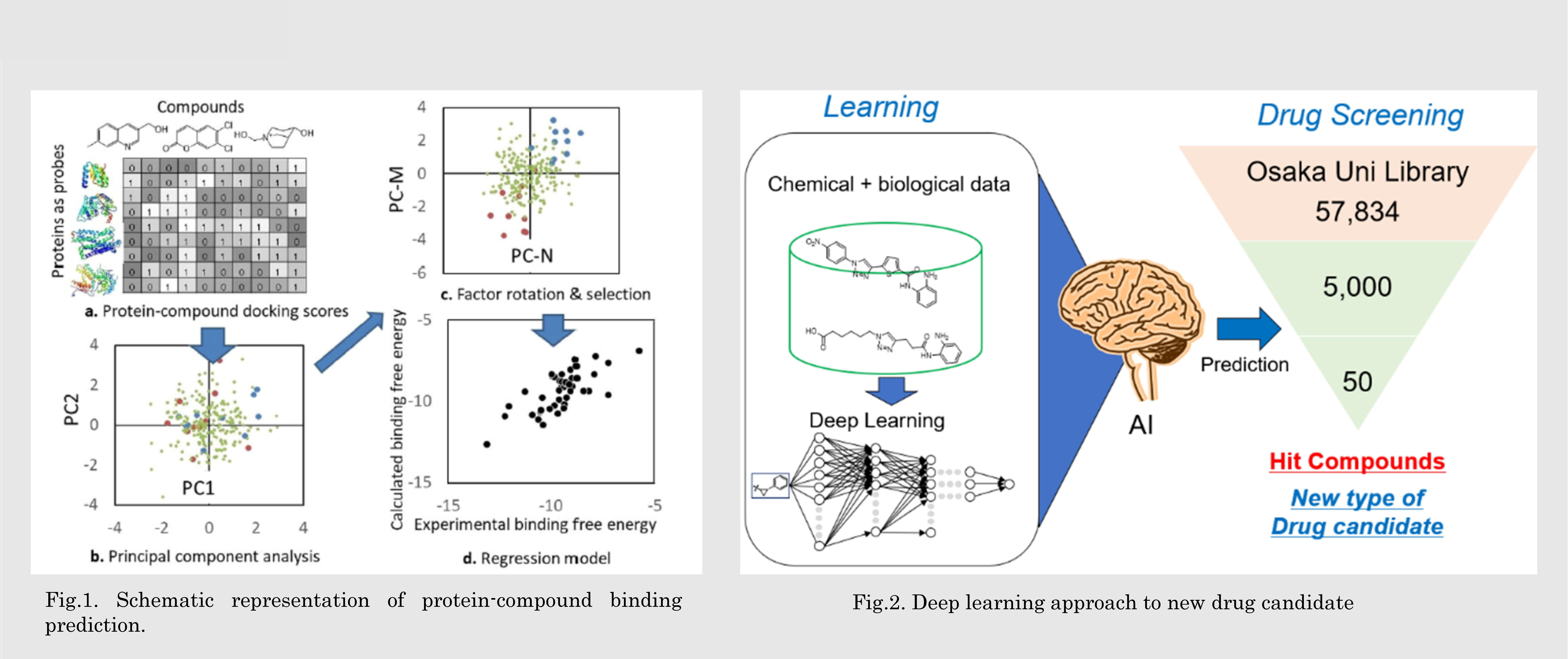
Fig.2. Deep learning approach to new drug candidate
文献 / Publications
ChemBioChem, 2020, 21, 1968.; Bioorg. Med. Chem., 2019, 27, 3339.; Synthesis, 2019, 51, 1178.; Mol. Inf., 2018, 37, 1700120.; Biosci. Biotechnol. Biochem., 2017, 81, 1279.; Heterocycles, 2017, 95, 370.; Synthesis, 2016, 48, 2191.; Chem. Pharm. Bull., 2016, 64, 961
研究者HP
- yyamashita
sanken.osaka-u.ac.jp
- https://www.sanken.osaka-u.ac.jp/labs/cmc/yasunobu-yamashita/